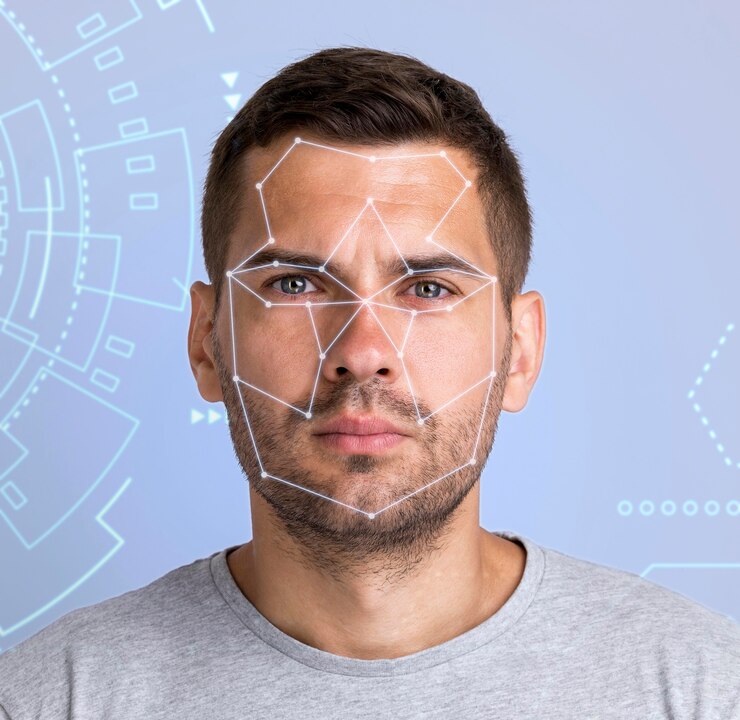
Faces are multidimensional visual stimuli and provide a variety of information such as individual identity, gender, age, race, mood and intentions. Facial recognition is a biometric method that is quite popular. Faces are more difficult to imitate, modify or steal compared to keys or passwords for non-biometric security. One artificial neural network method that can be used for facial recognition is the Extreme Learning Machine. Facial expressions can be divided into 7, namely happy, sad, angry, disgusted, afraid, surprised and neutral. The testing process in this study used 150 facial images for training data and 60 facial images for test data. Testing was carried out using 3 choices of parameters for the number of hidden neurons, namely 10, 30 and 50. As well as 5 image condition parameters, namely normal, expressive, face direction, dim lighting and distance to the webcam. The results of the system’s accuracy in facial recognition are 90% using 50 hidden neurons. These characteristics can be seen from physical characteristics, such as fingerprints, facial expressions, eye retina and voice.
In Facial Recognition Using Extreme Learning Machine A facial analyzer is software that identifies or confirms a person’s identity using their face. The facial analyzer functions to identify and measure facial features in an image. Facial recognition can identify human faces in images or videos, determine whether faces in two images belong to the same person, or search for faces among a large collection of existing images. Biometric security systems use facial recognition to uniquely identify individuals during user onboarding or sign-in and strengthen user authentication activities. Mobile and personal devices also commonly use facial analysis technology for device security. Facial recognition can identify a person by comparing faces in two or more images and assessing the likelihood of facial matches. For example, this technology can verify that the face of a selfie taken from a cell phone camera matches the face in the image of a government-issued ID such as a driver’s license or passport, as well as verify that the face shown in the selfie does not match a face in a collection of previously taken faces.
In other research, Extreme Learning Machine (ELM) was used to modify CNN at the classification layer to overcome long training times. This research combines methods that have been previously researched by utilizing the advantages of each method. LTP imagery is used to solve lighting problems. Then, the LTP image is used as input to the CNN architecture for the feature extraction process. At the classification layer, the CNN is modified by removing the fully connected layer and replacing it with the ELM method. The purpose of this CNN modification is to overcome the time-consuming training on backpropagation. Neural networks-based approaches for facial recognition rely on techniques from machine learning with facial image characteristics. Conventionally, facial images use backpropagation validation. Literature Facial recognition is widely used in research as outlined in the review of face recognition as in previous research. Finding a method for face recognition that can significantly improve efficiency during the face detection process. Other research finds out which parts of the human face are more important to achieve a high level of accuracy with the help of convolutional neural networks.
“For friends who want to learn more deeply about the world of technology and other information, you can stop by the best informatics and information systems study program at Alma Ata University, Yogyakarta”